Complete ‘How To’ Guide: Master Data Management Framework and Strategy for Effective Data Governance in 2023

Master Data Management Framework and Strategy for Effective Data Governance
Businesses and organizations rely on making informed decisions using data to gain a competitive advantage in the current data-driven world. However, as data volume, velocity, and variety continue to increase, managing and governing data effectively has become a daunting task. This is where Master Data Management (MDM), a framework that provides a structured approach to managing and governing critical data assets across an organization, plays a crucial role.
Master Data Management is not only a technology solution but a comprehensive set of processes, policies, and tools that enables organizations to manage and maintain accurate, consistent, and reliable data. MDM helps organizations to create a single source of truth for critical data, such as product, financial, employee, customer data, and other master records that are sharable across the organization and leveraged for various business processes and analytics.
MDM Framework
MDM framework is a comprehensive approach that enables organizations to manage and maintain their critical business data efficiently. MDM framework provides a systematic way to standardize and synchronize data across the organization, enabling stakeholders to make informed decisions based on accurate, timely, and consistent data. In this section, we will explore the definition, benefits, and challenges of implementing an MDM framework, helping organizations build a solid foundation for an effective master data management plan.
Components of MDM framework
The Master Data Management (MDM) framework comprises various components that enable organizations to manage their master data effectively. Some of the essential components:
Data Governance
Data governance is a critical component of an MDM framework. It involves defining policies and procedures for managing data, ensuring data quality, and enforcing data standards. This component is crucial for establishing a governance structure that enables stakeholders to manage data effectively.
Data Integration
Data integration involves collecting and combining data from different sources to create a unified view of the business-critical data. MDM solutions must have robust data integration capabilities to ensure data accuracy, consistency, and completeness across all enterprise applications.
Data Quality
Data quality involves defining data quality standards and ensuring that data meets those standards. This component is essential for ensuring data is accurate, complete, consistent, and meets business needs.
Data Modeling
Data modelling involves defining data entities, attributes, and relationships. This component is critical for ensuring that data is organized and structured to make it easy to manage and use.
Metadata Management
Metadata management involves managing the information that describes data. This component describes reference data and is essential for ensuring that stakeholders can find and understand data easily.
Master Data Repository
The master data repository is the central location where organizations store their master data. This component is critical for ensuring stakeholders can access the latest data version.
Data modelling and data governance
Data modelling and governance are two critical components of a Master Data Management (MDM) framework. Data modelling involves defining the structure and relationships of data entities, attributes, and tables. On the other hand, data governance involves defining policies and procedures for managing data, ensuring data quality, and enforcing data standards. Data modelling and governance work together to ensure that data is managed effectively.
MDM architecture
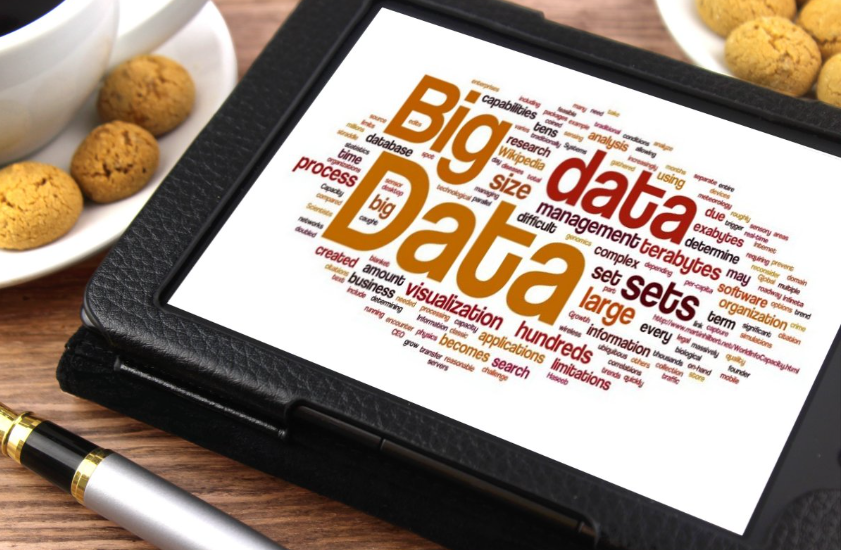
Master Data Management architecture is a system of technologies, processes, and policies that help organizations to manage their critical business and manage master data together. MDM solutions provide a single, authoritative source of truth for all master data entities across the organisation, such as customers, products, suppliers, and employees.
MDM architecture typically consists of a hub-and-spoke model, where a centralized MDM hub is the primary repository for all master data. The MDM hub connects multiple external data sources through various connectors, such as web services, APIs, or ETL (extract, transform, load) tools. The hub also serves as the integration point for data cleansing, enrichment, standardization, and validation.
MDM architecture can be deployed in different ways, such as on-premise, cloud-based, or hybrid. Each deployment model has its own benefits and trade-offs, depending on the organization’s specific needs and requirements.
Hierarchy management and data quality
Hierarchy management is an important aspect of MDM that involves creating and maintaining a hierarchical structure for data elements. This structure helps in organizing data into meaningful categories and subcategories and also establishes relationships between data elements. Hierarchy in master data management solution is particularly important for businesses that deal with large volumes of complex data, as it provides a way to easily navigate and search through the data.
Data quality is another critical component of MDM, as it ensures that the system’s data is accurate, complete, consistent, and up-to-date. Poor data quality can have serious consequences for businesses, leading to errors, delays, and inefficiencies in decision-making processes. Data quality can be improved through various methods, such as data profiling, cleansing, standardization, and enrichment. These techniques help to identify and eliminate duplicate, inconsistent, and inaccurate data and fill in any missing or incomplete information.
In order to maintain high levels of data quality, MDM solutions often incorporate data governance processes and workflows. These processes establish rules and guidelines for data management, and provide mechanisms for monitoring and enforcing compliance with these rules. Data governance helps to manage data consistently and effectively across the organization, and also provides a way to track changes and maintain an audit trail of data lineage. This is important for regulatory compliance, as well as for maintaining trust and transparency with customers and stakeholders.
Metadata management and data security
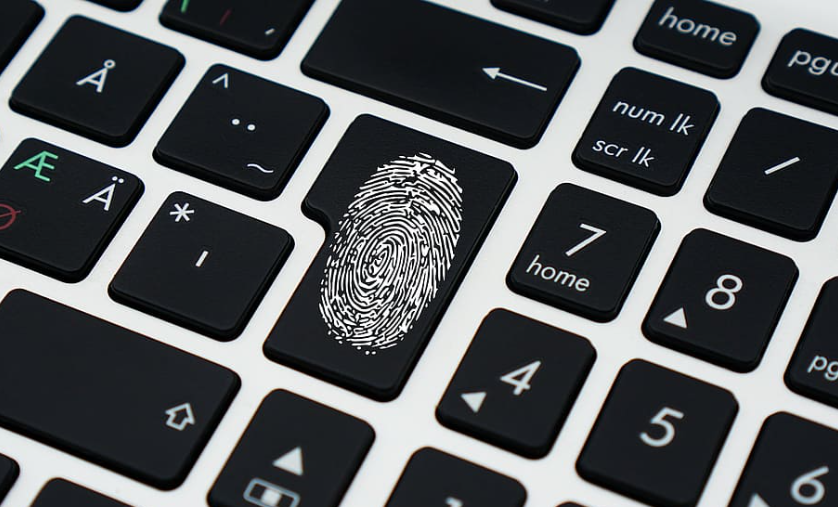
Metadata management is a critical aspect of any MDM strategy. It involves managing the information about your organisation’s data, including its source, structure, format, and usage. Metadata management ensures that data is properly identified, catalogued, and classified, making it easier to find, access, and use. It also helps ensure data is used consistently across the organization, reducing errors and improving decision-making.
Data security is another essential component of MDM. It involves protecting the data from unauthorized access, modification, or deletion. Data security includes measures such as authentication and access control, encryption, and data backup and recovery. It also ensures data complies with regulations and industry standards, such as GDPR and PCI DSS.
To effectively manage metadata and ensure data security, MDM architecture should incorporate features such as:
Metadata repositories
A central repository for metadata can help manage metadata consistently across the organization. It should be accessible to all stakeholders who need to use it.
Data lineage
Data lineage allows you to track the flow of data from its source to its destination. It helps ensure data integrity, accuracy, and reliability.
Role-based access control
This feature allows you to control who can access data based on their job roles and responsibilities.
Encryption
Encryption is a process that scrambles data to make it unreadable to unauthorized users. It ensures that data remains confidential, even if it falls into the wrong hands.
Data backup and recovery
Data backup and recovery ensure that data can be restored if it is lost, damaged, or stolen. It helps ensure business continuity and reduces the risk of data loss.
Best practices for MDM framework
A robust MDM framework requires proper planning and implementation. Here are some best practices that can help in achieving an effective MDM solution:
Clearly define business objectives and goals
Before implementing an MDM solution, defining clear business objectives and goals is essential. This will help to ensure that the MDM solution aligns with the organization’s goals and provides the desired outcomes.
Establish data governance policies
Data governance policies are essential for ensuring that data is managed effectively and consistently across the organization. This includes establishing data ownership, data quality standards, data security policies, and data privacy policies.
Develop a data quality strategy
A data quality strategy should be developed to ensure that data is accurate, complete, and consistent. Data quality tools can be used to automate data cleansing, profiling, and monitoring.
Implement a scalable architecture
MDM solutions should be designed to be scalable and adaptable to accommodate future growth and changing business requirements. It is essential to choose an MDM solution that can scale and integrate with other systems and applications.
Leverage metadata management
Metadata management is critical for managing and understanding data. Metadata provides context and meaning to data, which is essential for effective data management. A metadata management strategy should be developed to ensure that metadata is captured, stored, and utilized effectively.
Implement data security and privacy measures
MDM solutions must include robust data security and privacy measures to protect sensitive and confidential data. Access controls, encryption, and data masking can be used to secure data and ensure compliance with regulatory requirements.
Establish a data stewardship program
Data stewardship is critical for ensuring that data is managed effectively across the organization. A data stewardship program should be established to define roles and responsibilities for managing data and ensure that data is managed effectively.
Master Data Management Strategy
Importance of MDM strategy
The importance of an MDM strategy cannot be overstated as it provides a roadmap for the successful implementation and maintenance of an MDM solution. The following are some reasons why an MDM strategy is crucial:
Provides clarity
An MDM strategy helps to establish a clear understanding of the goals and objectives of the MDM initiative, the scope of the project, and the roles and responsibilities of the team members involved.
Reduces duplication and redundancy
With an MDM strategy in place, organizations can avoid the pitfalls of data duplication and redundancy that often result from a lack of centralized data management.
Enables data governance
An MDM strategy enables organizations to implement a robust data governance program, which ensures that data is accurate, consistent, and up-to-date, and helps to mitigate risks associated with data breaches and regulatory compliance.
Increases efficiency and productivity
By providing a centralized view of data, an MDM strategy can help organizations reduce the time and effort required to access, analyze, and report on data, enabling employees to make better-informed decisions in less time.
Supports digital transformation
An MDM strategy can help organizations transform their operations by providing the foundation for advanced analytics, machine learning, and other emerging technologies that require high-quality data.
Creating an MDM roadmap
Creating an MDM roadmap is essential for successfully implementing an MDM strategy. The following are some key steps involved in creating an MDM roadmap:
Establish goals and objectives
The first step in creating an MDM roadmap is establishing clear goals and objectives. These goals and objectives should align with the overall business strategy and be specific, measurable, achievable, relevant, and time-bound.
Define scope
Once the goals and objectives have been established, it is important to define the scope of the MDM initiative. This includes identifying the data domains that will be included in the MDM program and the stakeholders who will be impacted by the initiative.
Assess current state
The next step is to assess the organisation’s current state of data management. This includes identifying the current data management processes, the data governance framework, and the existing technology infrastructure.
Identify gaps
Based on the assessment of the current state, it is important to identify the gaps that exist in the current data management practices. This includes identifying areas where data quality is poor, where data is not well integrated, and where there are redundancies or inconsistencies in data.
Develop a data model
Once the gaps have been identified, the next step is to develop a data model. This involves identifying the key data entities that need to be managed, as well as the relationships between these entities.
Determine technology requirements
With the data model in place, the next step is determining the MDM initiative’s technology requirements. This includes identifying the technology infrastructure needed to support the data management processes and the tools and applications required for data integration, data quality, and data governance.
Develop a roadmap:
The next step is to develop a roadmap for the MDM initiative based on the technology requirements. This should include a detailed plan for implementing the MDM program and a timeline for the various stages of the initiative.
Implement and monitor
Once the roadmap has been developed, it is important to implement the MDM program and monitor its progress. This includes establishing metrics to measure the initiative’s success and conducting regular reviews to identify any areas where improvements can be made.
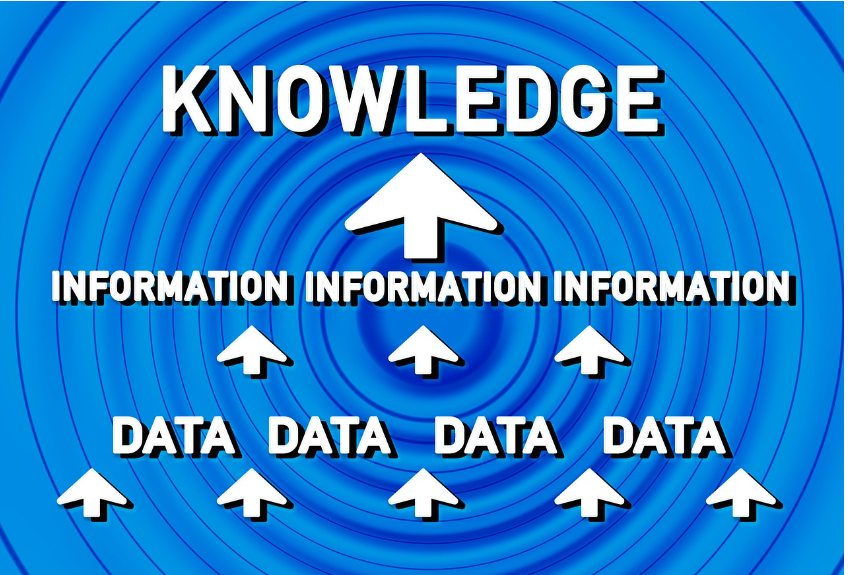
Aligning MDM strategy with business goals
Aligning MDM strategy with business goals is crucial for ensuring that the MDM initiative delivers value and aligns with the overall business objectives. To achieve this alignment, the MDM strategy should be developed in close collaboration with business stakeholders to ensure that it addresses the key business challenges and opportunities.
The following are some of the key considerations for aligning MDM strategy with business goals:
Business objectives
The MDM strategy should be aligned with the organisation’s overall business objectives. This involves understanding the business goals, challenges, and opportunities and developing an MDM strategy that can help the organization achieve its goals.
Data requirements
The MDM strategy should be developed based on an understanding of the organisation’s data requirements. This involves identifying the critical data elements required to support key business processes and ensuring that the MDM initiative effectively manages these data elements.
Business process alignment
The MDM strategy should be developed in close collaboration with business stakeholders to ensure that it aligns with the organization’s key business processes. This involves understanding how data flows through the organization and identifying the key touchpoints where data quality and consistency are critical.
Data governance
The MDM strategy should be developed in the context of the organization’s data governance framework. This involves ensuring that the MDM initiative aligns with the organization’s data governance policies and procedures and that the MDM team works closely with the data governance team to maintain data quality and consistency.
IT alignment
The MDM strategy should be developed in close collaboration with the IT organization to ensure it aligns with the overall IT strategy and architecture. This involves ensuring that the MDM initiative leverages existing IT infrastructure and tools where possible and that it aligns with the organization’s overall data management and integration strategy.
MDM governance and stakeholder management

MDM governance and stakeholder management are crucial components of a successful MDM initiative. Governance refers to the policies, procedures, and processes that ensure the proper management of data across the organization. Stakeholder management involves identifying the key stakeholders in the MDM initiative, understanding their needs, and effectively communicating with them to ensure their buy-in and support.
Stakeholder management is equally important in ensuring the success of MDM initiatives. Key stakeholders can include business leaders, IT professionals, data analysts, data stewards, and end-users. Each of these groups has unique requirements and perspectives that need to be considered when developing and implementing an MDM strategy. Effective stakeholder management involves engaging with these groups throughout the MDM initiative, communicating the benefits of the initiative, and addressing their concerns and feedback.
Organizations should establish a governance board that includes key stakeholders from across the organization to oversee the MDM initiative, ensure compliance, and make decisions regarding data management. Regular communication with stakeholders is also critical, and organizations should establish regular feedback mechanisms to ensure that stakeholders are engaged and informed throughout the MDM initiative.
Benefits of MDM Framework and Strategy
Improved data quality and consistency
Improved data quality and consistency is a critical benefit of implementing an MDM strategy. By establishing a centralized system for managing data, an organization can ensure that all data is accurate, complete, and consistent across all systems and applications. This can help prevent errors and inconsistencies arising when data is managed in silos or different systems.
An MDM framework creates data standards, such as naming conventions, data definitions, and data formats, and ensures that these standards are consistently applied across all systems and applications.
Increased efficiency and productivity
An effective MDM framework and strategy can lead to increased efficiency and productivity in many ways. By providing a single, authoritative source of data, MDM reduces the time and effort spent on manually reconciling conflicting information from multiple sources. This eliminates duplicate efforts, reduces errors, and frees up resources that can be used for other tasks.
MDM also enables faster and more accurate data processing and analysis, allowing businesses to make more informed decisions and respond more quickly to changing market conditions. With a comprehensive understanding of their data, businesses can identify areas of inefficiency and target them for improvement.
In addition, MDM can streamline business processes and automate data-related tasks, reducing the need for manual intervention and improving the speed and accuracy of operations. This can lead to significant time and cost savings over the long term. Overall, increased efficiency and productivity are key benefits of a well-designed and implemented MDM framework and strategy.
Enhanced data security and compliance
Enhanced data security and compliance is another crucial benefit of implementing an MDM framework and strategy. With MDM, you can ensure that sensitive information is protected, and data privacy regulations are followed. A well-implemented MDM solution can help identify and mitigate risks related to data breaches, unauthorized access, or data misuse.
MDM helps in controlling access to sensitive information by implementing role-based access controls and defining access policies. It also helps in maintaining an audit trail of data usage, providing transparency into data access and usage. This information can be helpful in demonstrating compliance with regulatory requirements such as GDPR, HIPAA, or CCPA.
In addition, MDM can help in ensuring data accuracy and consistency, reducing the risk of errors and inconsistencies in reporting or compliance requirements. By implementing data governance policies and data quality rules, organizations can ensure that data is reliable and meets the required standards. Overall, enhanced data security and compliance can provide organizations with a competitive advantage and build trust with customers and stakeholders.
Better decision-making and business outcomes
Implementing an MDM framework and strategy can lead to better decision-making and improved business outcomes. By having accurate and consistent data, organizations can make informed decisions based on reliable information. This can result in increased customer satisfaction, improved operational efficiency, and better financial performance.
Additionally, MDM can help organizations identify new revenue opportunities, reduce costs, and mitigate risks. With a clear understanding of their data, organizations can make informed decisions that align with their strategic goals and drive overall success.
Challenges in Implementing MDM Framework and Strategy
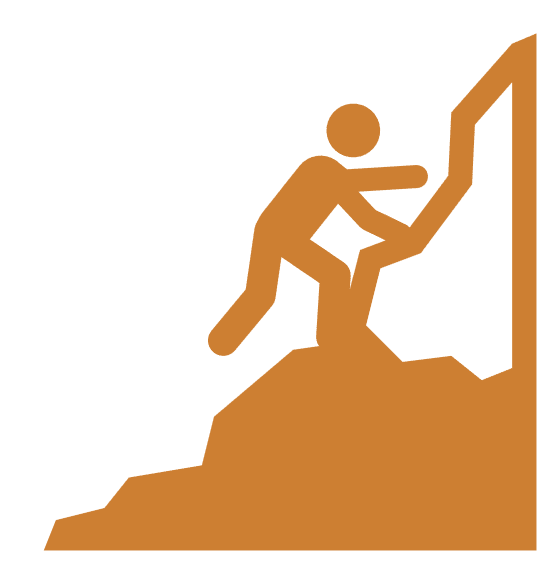
Data silos and legacy systems
Data silos and legacy systems can pose significant challenges in implementing an MDM framework and strategy. Many organizations have data stored in disparate systems that are not integrated or easily accessible, resulting in data silos that can hinder effective decision-making and business operations. Legacy systems can also be difficult to integrate with newer technologies and systems, creating additional challenges in data management.
Data silos and legacy data systems can lead to duplicate data entry, inconsistent data, and inaccurate data, ultimately leading to poor decision-making and negative business outcomes. They can also make it difficult to maintain data security and compliance, as data may be stored in multiple locations with varying levels of access and security.
To overcome these challenges, organizations must develop a comprehensive plan for data integration and migration from legacy systems to the new MDM framework. This plan should include identifying and prioritizing critical data sources and systems, defining data governance policies, and establishing a phased approach for implementation of master data solution. Additionally, it is important to engage all stakeholders in the process, including IT teams, business leaders, and end-users, to ensure buy-in and successful adoption of the new MDM strategy.
Data governance and ownership
Data governance and ownership is another significant challenge that organizations face while implementing an MDM framework and strategy. Data is often created and maintained by multiple departments or business units, leading to data ownership and accountability issues. This can result in data inconsistencies, conflicts, and inaccuracies, leading to poor data quality and negatively impacting business decisions.
Furthermore, data governance is critical to ensuring compliance with data privacy regulations and maintaining data security. Without proper data governance, there is a risk of data breaches, non-compliance with regulations, and legal consequences.
To overcome these challenges, it is essential to establish clear data governance policies and procedures that define data ownership, access controls, data quality standards, and compliance requirements. Organizations must also establish data stewardship roles and responsibilities to ensure that data is managed and governed effectively across the organization. Additionally, establishing a data governance council or committee can help facilitate collaboration and decision-making around data governance issues.
Data privacy and regulations
Data privacy and regulations are other significant challenges in implementing an MDM framework and strategy. With the growing concerns about data privacy and the increasing number of regulations globally, organizations need to ensure that their data management practices comply with various regulations, such as the General Data Protection Regulation (GDPR) and the California Consumer Privacy Act (CCPA).
The MDM framework should have built-in features that help ensure data privacy compliance, such as consent management and the ability to handle personal data deletion requests. In addition, organizations must establish policies and processes for handling sensitive data, such as personal information and financial data.
Change management and stakeholder buy-in
Change management and stakeholder buy-in are critical challenges in implementing an MDM framework and strategy. MDM requires significant organizational change, including changes in processes, roles, and responsibilities. This can be challenging to manage, particularly when stakeholders resist change. Therefore, it is essential to have a clear communication plan and engagement strategy in place to ensure that stakeholders are aware of the benefits of MDM and are willing to participate in the implementation process.
A lack of stakeholder buy-in can also lead to a lack of funding and resources for the MDM project, making it challenging to implement the necessary changes. Change management involves identifying and engaging with key stakeholders to get their buy-in for the project. This includes addressing their concerns, ensuring they understand the benefits, and getting their input into the project planning process.
Furthermore, MDM implementation is a continuous process, and stakeholders must understand that their participation is required beyond the initial implementation stage. Therefore, it is essential to establish ongoing communication and engagement channels with stakeholders to ensure continued support and participation in the MDM program. Organizations can successfully implement and maintain an MDM framework and strategy by addressing change management and stakeholder buy-in challenges.
Wrap Up
In conclusion, mastering data management through implementing a well-designed MDM framework and strategy is essential for organizations seeking to maximize the value of their data assets. With the ever-growing amount of data generated and collected by businesses, MDM has become a crucial factor in ensuring data quality, consistency, and security.
As organizations continue to recognize the importance of MDM, there are some future trends to keep in mind. These include the rise of cloud-based MDM solutions, the integration of artificial intelligence and machine learning into MDM processes, and the increasing adoption of blockchain technology for data governance and ownership.
It is clear that MDM is not just a one-time implementation but an ongoing process that requires constant attention and refinement. Therefore, it is important for organizations to commit to adopting an MDM framework and strategy and invest in the necessary resources to ensure its success.
In light of the benefits of MDM and the potential for future trends in the field, organizations should take a proactive approach and prioritize the implementation of an MDM framework and strategy. By doing so, they can unlock the full potential of their big data assets and gain a competitive advantage in their respective industries.
Phot credit – Credit: Ranjbar.hani, CC BY-SA 4.0 https://creativecommons.org/licenses/by-sa/4.0, via Wikimedia Commons.
Article also published on author’s Medium.com profile
More from the author on RTE, Agile coach, Scrum Master / Product Owner / Why you need a Career Coach / Interview Coach / Managing Managers / IT Career switch. Ilam also is a keen AI enthusiast & writes about popular AI tools like Jasper, Writecream etc.